Our lab members recently presented in the recent LabPhon19 conference, held from June 27th to 29th, 2024, in Seoul, South Korea. The conference theme was “Where speech sounds meet the architecture of the grammar and beyond.” We presented findings from three distinct areas of investigation: phonetic substance in language learning, tonal representation in Hakka dialects, and the influence of naturalness bias on phonological variation.
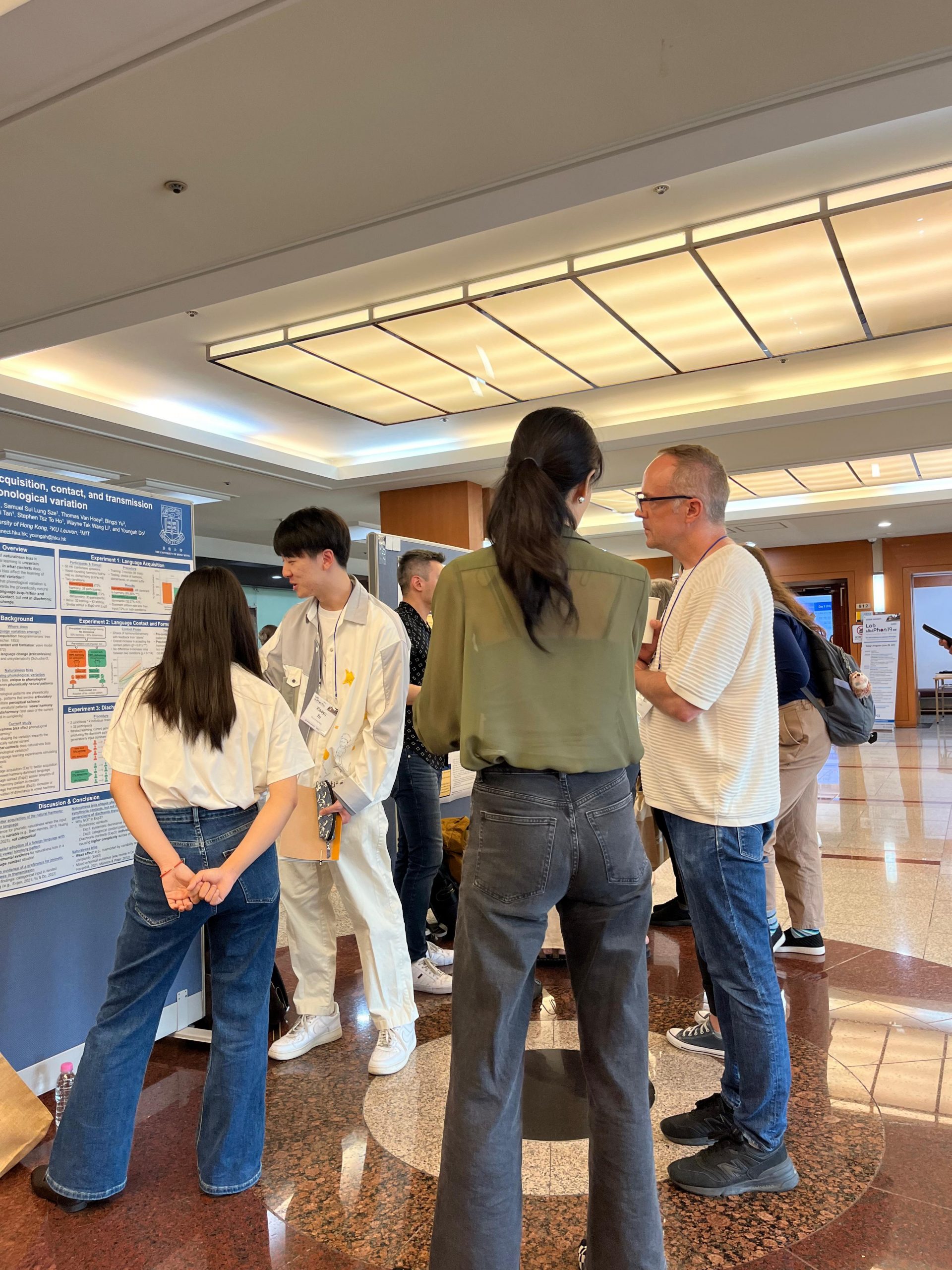
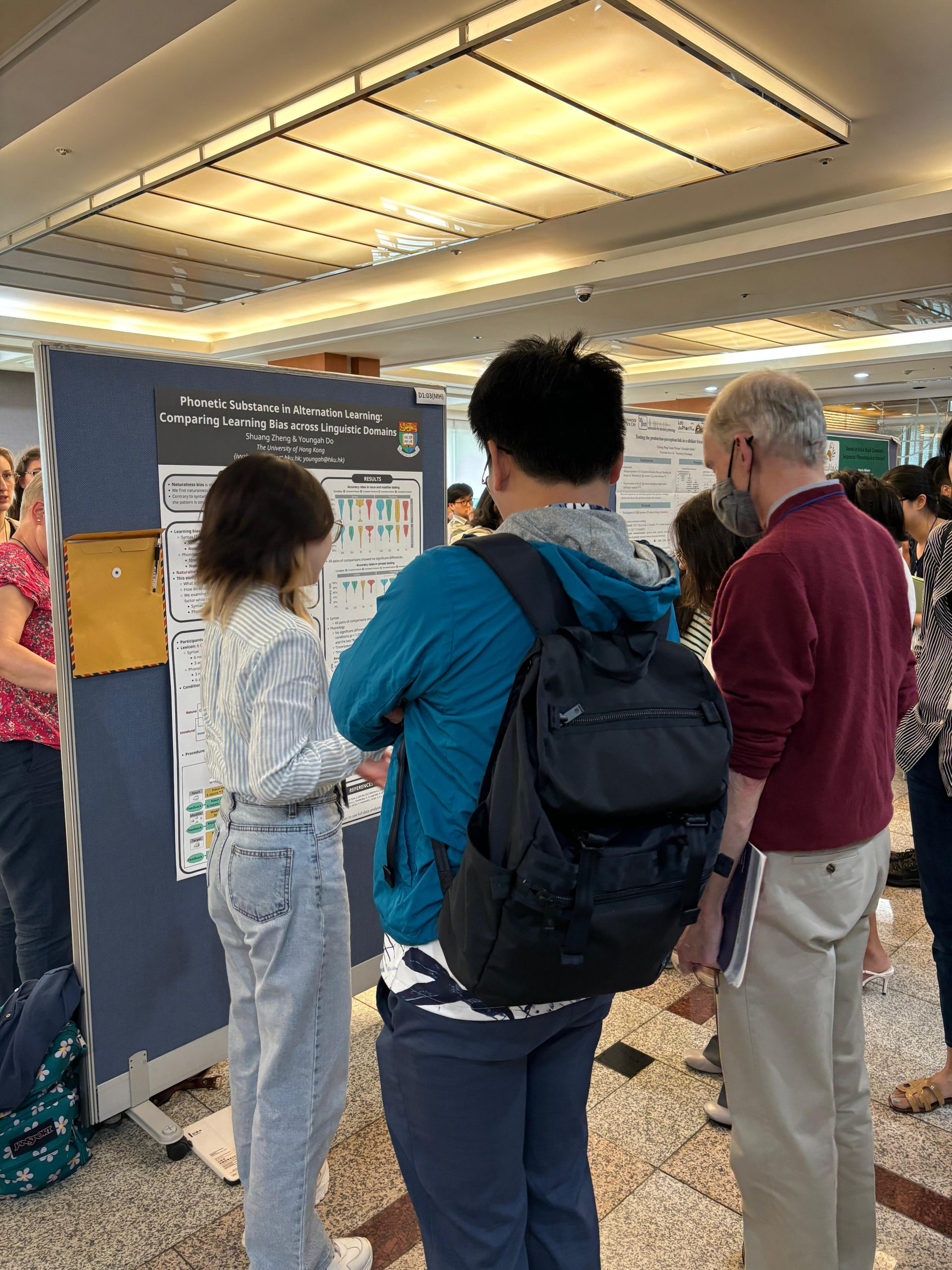
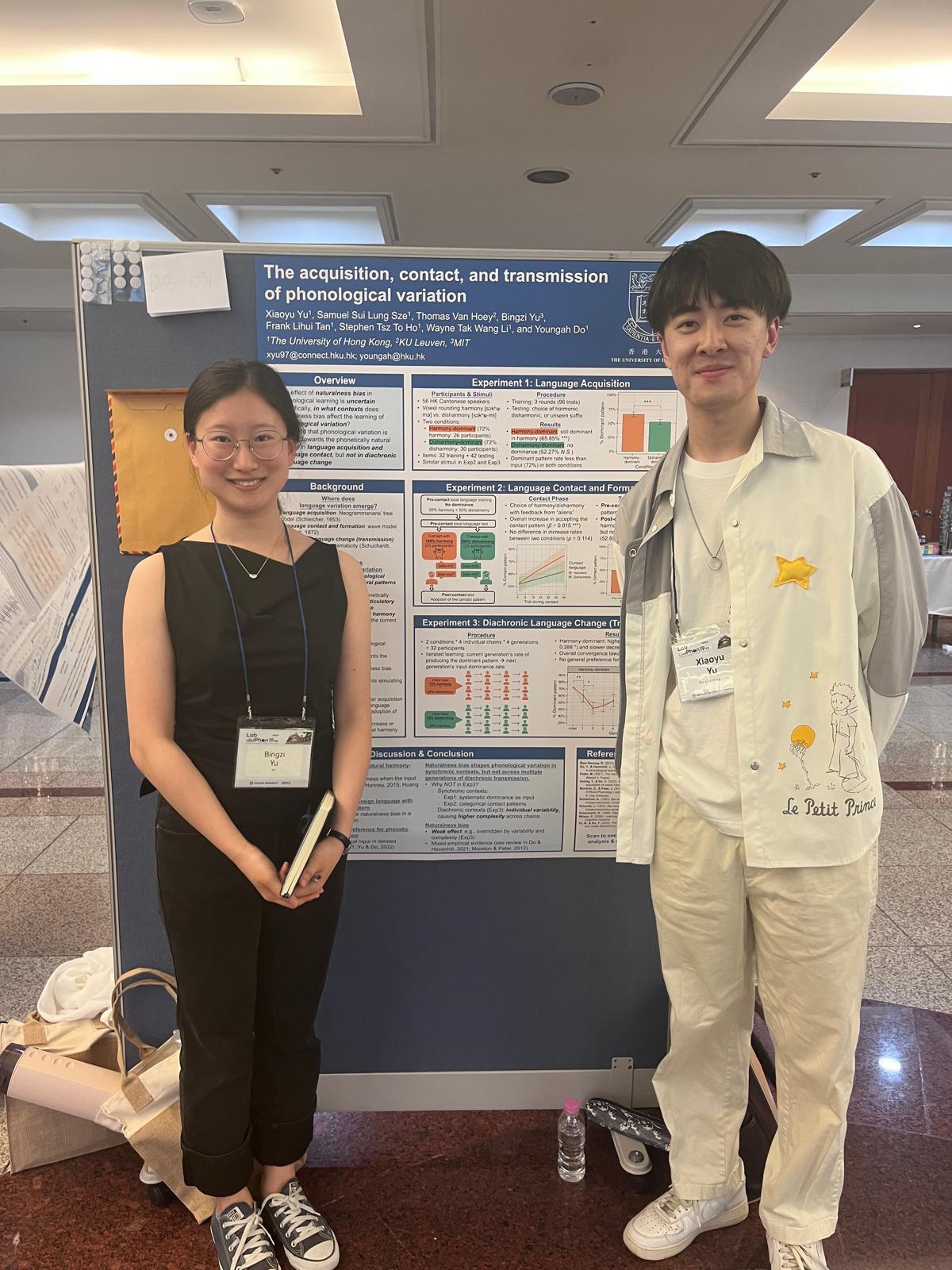
Poster Presentations
- Phonetic substance in alternation learning: This study by Ivy and Youngah investigated how learners acquire grammatical and sound patterns in different domains. The results suggest that while both domains involve structural complexity and naturalness as learning biases, these biases play a stronger role in phonological learning, particularly when the target pattern is complex and unnatural.
- Syllable-based or Word-based? Representation of tones undergoing merger in Hakka: Ming and Jon explored how native speakers of Wangmudu Hakka represent tones in their minds. Their findings suggest that for tones undergoing merger, speakers rely on word-level representations rather than syllable-level or generalized sandhi rules.
- The acquisition, contact, and transmission of phonological variation: Xiaoyu, Samuel, Thomas, Bingzi, Frank, Stephen, Wayne and Youngah examined how biases influence phonological variation learning in different language learning contexts. Their results suggest that a bias towards phonetically natural patterns guides learning in acquisition and contact situations, but not necessarily during language transmission.
Corpus Workshop Presentation
- Attention-LSTM Autoencoder for Phonotactics Learning from Raw Audio Input: Frank and Youngah presented a study on how a neural network model can learn phonotactic knowledge from raw audio data. Their model, designed to mimic early stages of infant language learning, successfully captured the influence of surrounding sounds on the pronunciation of stops following a sibilant fricative in English.
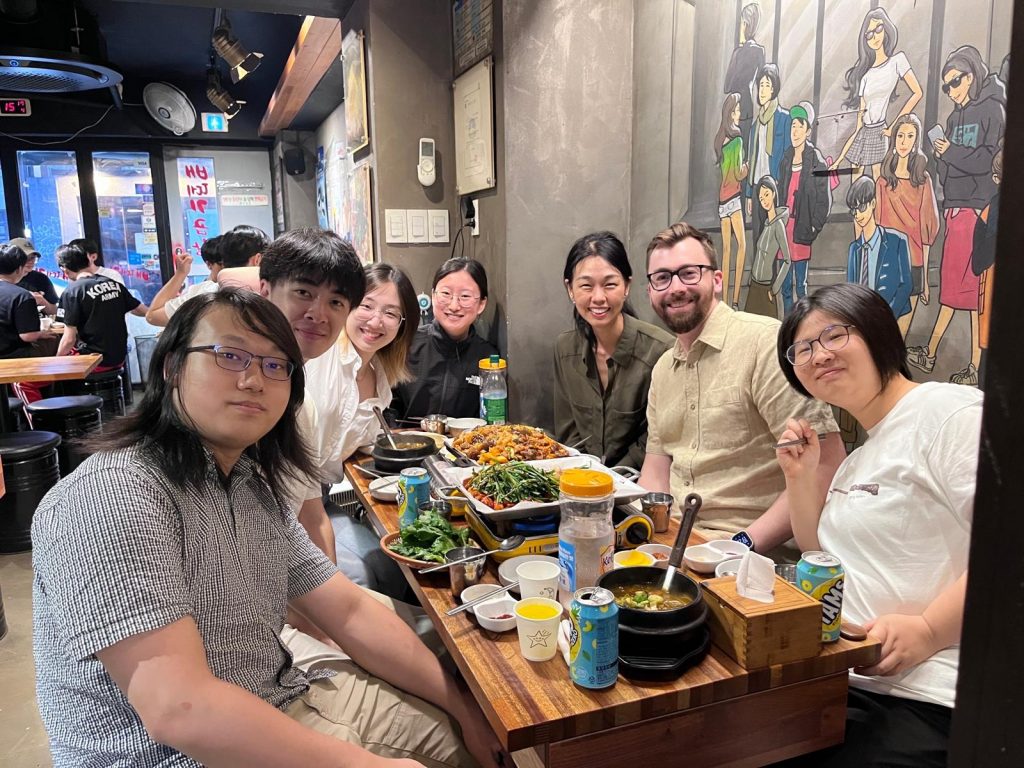